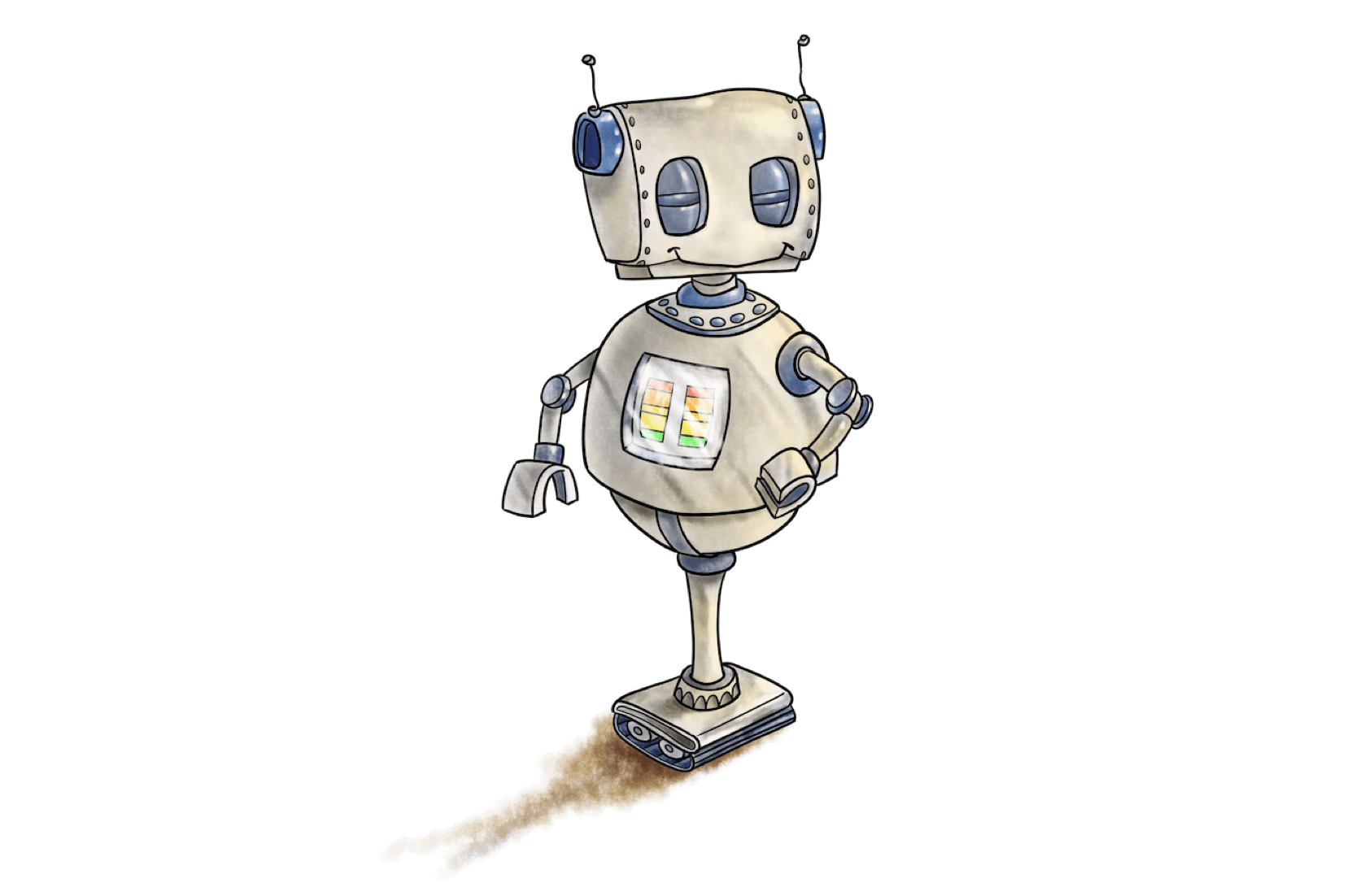
Here’s something to think about. What do all of the following have in common?
Siri. Netflix. Self-driving cars. Predictive text. Face recognition. Spotify weekly playlists. Spam e-mail classifiers…
They’re all backed by bots. Clever bots that learn by having us teach them, without us even realising it.
Machine learning is an important arm of Artificial Intelligence that involves programming computers to use the information we give them to make predictions and decisions. As this process goes on, we teach them what we like, and how we respond to situations. And the longer we do this, the smarter the algorithms become, until little human intervention is needed.
The sheer amount of information every single person connected to the internet, smartphones and transport systems (so, every person alive) create daily translates into some pretty hefty data sets. And these data sets are at the core of machine learning algorithms.
We’re not quite talking about a 2001-esque HAL powering the education system just yet (although with Google’s recent advances in lip-reading AI, we may be getting close) machine learning is making some major changes across basically every industry.
It all may seem pretty futuristic, but historical data has been used widely to inform future predictions for a long time. Historical medical records to discover which patients should get what treatment, traffic data to reduce congestion, crime data to allocate the right amount of police to a place at specific points in time. So it’s not too far a stretch to apply it to an education system that’s crying out for change.
Traditional learning requiring a great deal of effort from us mere mortals. There are so many people involved in the process — course creators, instructors, learners. Learning professionals have a massive job in terms of creating content, monitoring results and providing the best feedback possible.
Learners, on the other hand, are unfortunately dealt an even shorter straw. A ‘one-size-fits-all’ approach is pretty standard in most contexts. You respond better to audio and visual learning? Aren’t too crash at remembering large sets of information? A lot of the time, it’s tough luck.
Luckily for both learners and instructors, recent advances in machine learning are opening up new opportunities to lift the barriers that have so long held down the individuality that the learning process needs. Artificial intelligence, psychology and education theory can inform the collection of data sets and analyse the data learners generate when they interact with a digital learning system.
Meet Cluey.
Cluey is a clever bot. He lives to serve one purpose only: to make the learning process more adaptive, responsive and personal everyday. He’s only just begun learning, but he’s already becoming pretty darn good at it.
Let’s suppose his learner is on the train on the way to work.
She’s using her team’s new learning app to complete a compliance program, and is about to answer a few of her questions for the day. She answers some quickly, while others take a little more time. Over the course of just one day, he’s taken in her answers and made some pretty important decisions about what to do the next time they meet.
The learning path will look a little different from now on. Why? Because Cluey has figured out that his learner had already mastered some of the topics she learnt about yesterday, and needs further attention in others, using her data to make decisions about what’s best for her learning. Clever.
This process will continue as long as the two are connected, and holds the potential to guide the development of learning not only for Cluey’s learner, but others too.
Think about how useful it would be to start a new training module or course, and being able to be fast-tracked to your skill and knowledge level just by answering a few questions! Less frustration, and less wasted time.
Where else is it already happening?
A few major breakthroughs that machine learning has had already had in education include:
- Grading systems that score work and detect plagiarism on large scales, automatically or through peer-grading (like Turnitin)
- Dynamic scheduling of student/teacher calendars
- Predictive analytics and data mining to improve learning, retention and application and to map the patterns of expert teachers
- Sensing learner engagement and using it to improve performance
- Content analytics to organise and optimise learning materials
The possibilities of machine learning are something we’re super excited about exploring at Yarno. However, there are a few challenges that need to be tackled.The collection of data and what happens to it afterwards is a question that leads to obvious privacy issues. The property rights of, the access to and the benefits of the data need to be clarified before its collection takes place. Once these concerns are addressed, the benefits can be taken advantage of with confidence.
Some challenges specifically related to learning that may arise include:
- Detailed inferences can't be made at a deep level until a considerable amount of data is relayed into the system. The use of robust benchmarking questions, as well as relevant past performance is a way of ensuring that the learner will receive the most accurate learning path
- The combination of online and offline learning could prove to be an issue in learner knowledge level, as it’s impossible for the system to learn from social and offline learning until it is tested online. Using a multifaceted system that measures both elements of learning may be a way to combat this issue.
- Predictions and changes to the learning path must be made immediately after the learner inputs a response. How frustrating would it be to answer a question correctly, then be presented with the same question the same day, or getting a question wrong and never having the chance to answer it again?
- Developing a machine learning model with a set of content that simultaneously accounts for diversity in both learner and content could present difficulties, and if not acknowledged correctly, this could lead to difficulty in comparison of results.
So how far will machine learning take education? Where will it end?
The value of machine learning and adaptive content in learning are multidimensional, and will become more pervasive and beneficial as the technology develops. Data will play a huge part in the way educational content is created, delivered and measured.
However, the relational element of learning needs to remain a priority. That’s where learning originated, and it’s a major part of how we learn best. Through empathy, experience and relationships.
Don’t let Artificial Intelligence freak you out. Technology isn’t an end to prevent human potential, but the tool that will help us achieve the best results possible.